“I know of scarcely anything so apt to impress the imagination as the wonderful form of cosmic order expressed by the ‘Law of Frequency of Error’,” the British polymath Francis Galton wrote in 1889. “The law would have been personified by the Greeks and deified, if they had known of it.”
Now, Galton may have had some pretty terrible opinions on a lot of things, but he was on the money here. So what is it about the Central Limit Theorem, as the “Law of Frequency of Error” is known today, that caused him to wax so lyrical?
What is the Central Limit Theorem?
If you’re not a mathematician or statistician, there’s a reasonable chance you won’t have heard of the Central Limit Theorem. You may, however, have come across a closely related concept: the “normal distribution”, sometimes known as the “bell curve”.
“[The] central limit theorem […] establishes the normal distribution as the distribution to which the mean (average) of almost any set of independent and randomly generated variables rapidly converges,” explained Richard Routledge, Professor of Statistics at Simon Fraser University, in an explainer for Britannica.
“[It] explains why the normal distribution arises so commonly,” he wrote, “and why it is generally an excellent approximation for the mean of a collection of data (often with as few as 10 variables).”
So, what does the theorem actually say? There are actually several different versions of it, and which one you choose will usually depend on how you’re approaching your particular problem. The most “standard” version, though, looks like this:
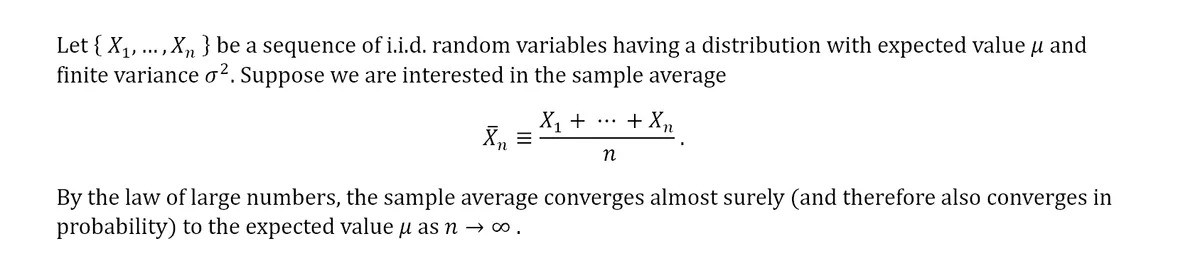
Or something like this. The CLT is unusually vibes-based for a mathematical theorem.
Image credit: IFLScience
Now, there’s obviously a lot to unpack there, but the general moral is this: under certain, relatively easy-to-achieve conditions, the probability distribution of some collection of variables will tend towards a normal distribution – that is, the iconic “bell curve” shape in which almost all outcomes are clustered around the mean, with probabilities dropping off the further away you move in either direction.
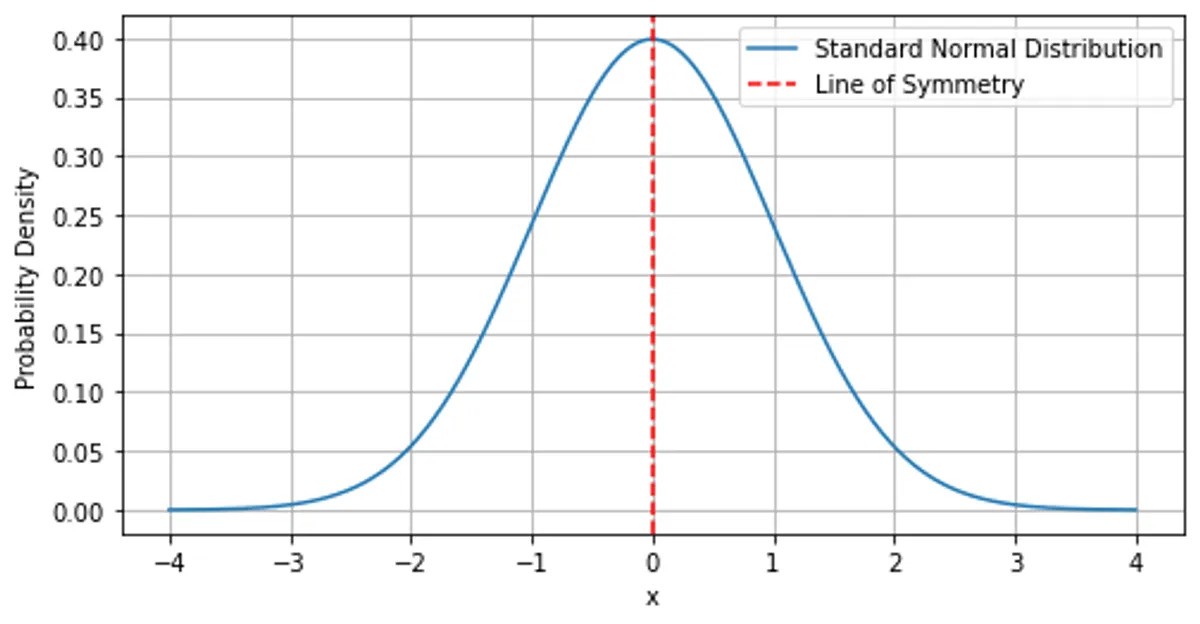
PSA: it will never look this perfect in real life without some finagleing.
“The setup is that we have a random variable, and that’s basically shorthand for a random process where each outcome of that process is associated with some number,” explained YouTuber Grant Sanderson in a video on the theorem for his channel 3Blue1Brown last year. “We’ll call that random number x.”
“The claim of the Central Limit Theorem is that as you let the size of that sum get bigger and bigger, then the distribution of that sum, how likely it is to fall into different possible values, will look more and more like a bell curve,” he said. “That’s the general idea.”
When can we use The Central Limit Theorem?
Let’s go back to the original statement of the theorem and check out some of those conditions. Now, we said they’re fairly easy to achieve, and that’s true – but that doesn’t mean they’re not important. So what are they?
Well, first, the variables being measured have to be i.i.d. – a shorthand for the mathematical term “independent and identically distributed”. Simply put, this means that each variable has to be mutually independent from all others, and all of them must have the same probability distribution.
If that explanation doesn’t help, let’s think of an example. Rolling a single dice is a good one: each outcome is unaffected by those preceding or following it, so they are independent, and each roll – not outcome, but roll; it actually doesn’t matter if the dice is weighted or not – has the same set of probabilities as every other roll, so they’re also identically distributed.
Alternatively, you can imagine a Galton board – a device invented by Galton specifically to demonstrate the Central Limit Theorem.
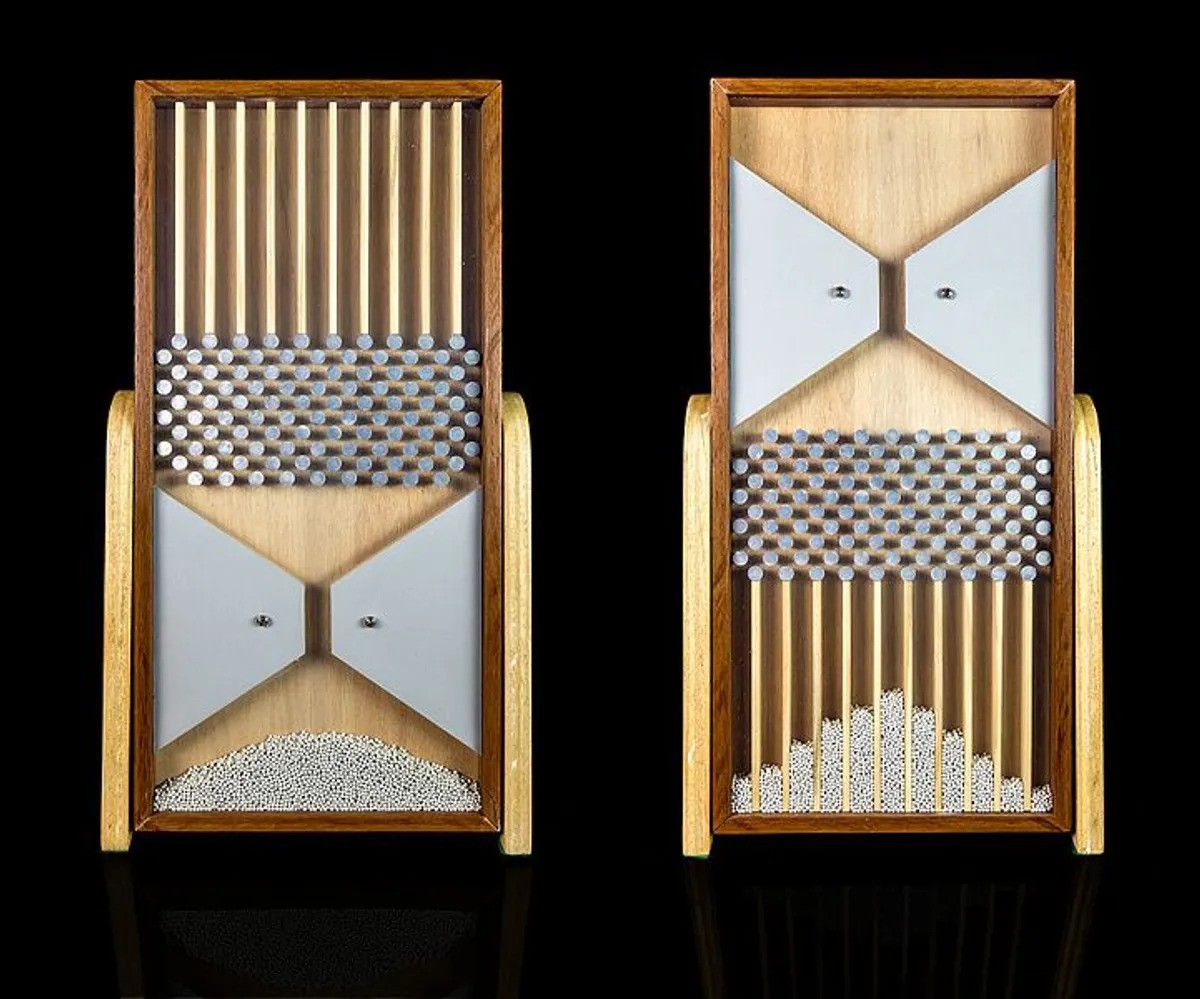
The original Galton board.
“Each bounce off the peg [in a Galton board] is a random process modeled with two outcomes,” noted Sanderson. “Those outcomes are associated with the numbers negative one and positive one […] What we’re doing is taking multiple different samples of that variable and adding them all together.”
“On our Galton board, that looks like letting the ball bounce off multiple different pegs on its way down to the bottom,” he explained, “and in the case of a die, you might imagine rolling many different dice and adding up the results.”
Why is the Central Limit Theorem useful?
So, let’s say we have our collection of i.i.d variables, and we’ve carried out enough trials to have a distribution resembling the normal. Why, you might ask, should we care about that?
In fact, the normal distribution tells us far more than just how many times an experiment resulted in a particular outcome.
“There’s a handy rule of thumb about normal distributions,” Sanderson explained, “which is that about 68 percent of your values are going to fall within one standard deviation of the mean, 95 percent of your values […] fall within two standard deviations of the mean, and a whopping 99.7 percent of your values will fall within three standard deviations of the mean.”
Now, there are a few ways in which this can be helpful to us. Say you’re starting a business making pants, and you want to decide the range of leg lengths you should include. You do some research, and find that the average height from floor to butt of men in the US is 88.74 centimeters (34.94 inches), with a standard deviation of 4.71 centimeters (1.85 inches). Neat.
That means that by making pants with leg lengths between 84.03 centimeters (33.1 inches) and 93.45 centimeters (36.8 inches), you can be pretty sure you’ll cover 68 percent of the market. Stretch that to a range of 79.32 centimeters (31.23 inches) and 98.16 centimeters (38.64 inches) and you’ll be reaching 95 percent of your potential customers.
Alternatively, we can go the other way. Say a friend tells you they rolled a dice 300 times, summed the outcomes, and got a total of 1,653. How likely is it that they’re lying?
Well, let’s consult the Central Limit Theorem. Dice rolls, as we’ve seen, are i.i.d., and so they should have an approximately normal distribution. A total of 1,653 means your pal was rolling an average of 5.51 with each roll – and according to the Central Limit Theorem, that’s almost entirely impossible. You should call bullshit.
It’s not just these constructed math-class problems that the theorem is useful for, either – it has serious real-world applications. “The central limit theorem […] plays an important role in modern industrial quality control,” Routledge wrote, as “the normal distribution is the basis for many key procedures in statistical quality control.”
“The first step in improving the quality of a product is often to identify the major factors that contribute to unwanted variations. Efforts are then made to control these factors,” he explained. “If these efforts succeed, then any residual variation will typically be caused by a large number of factors, acting roughly independently. In other words, the remaining small amounts of variation can be described by the central limit theorem, and the remaining variation will typically approximate a normal distribution.”
Order out of chaos
The Central Limit Theorem is, then, pretty ubiquitous throughout a range of industries – and for good reason. In a world inundated with data, it gives us a handy shortcut to understand the underlying patterns in random and disconnected information.
“It reigns with serenity and in complete self-effacement, amidst the wildest confusion,” rhapsodized Galton. “The huger the mob, and the greater the apparent anarchy, the more perfect is its sway.”
“It is the supreme law of Unreason,” he wrote. “Whenever a large sample of chaotic elements are taken in hand and marshalled in the order of their magnitude, an unsuspected and most beautiful form of regularity proves to have been latent all along.”